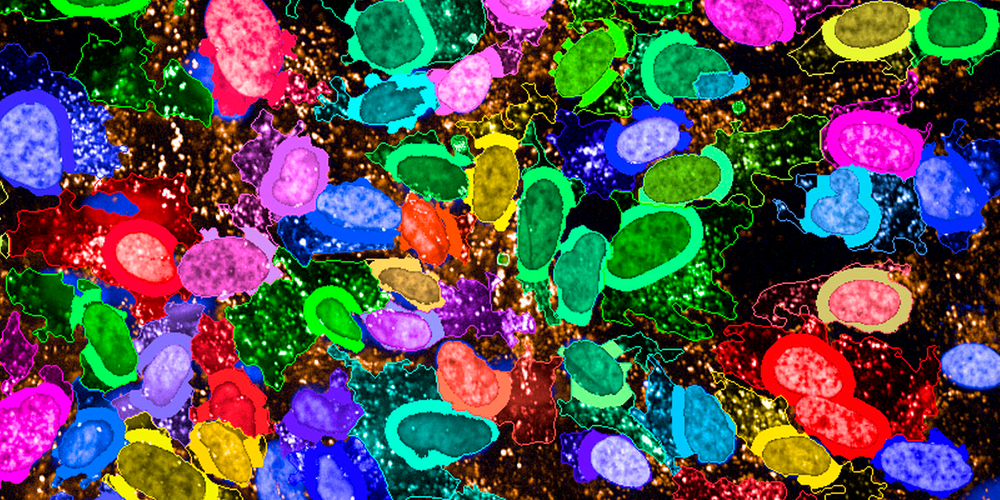
High-content assays can exhibit varying levels of complexity, but the advent of automated microscopy has created new opportunities for investigating a wide range of phenotypes. Image-based assays have proven useful in addressing numerous questions pertaining to cell biology. By utilizing microscopy-based screens, it is possible to accurately measure phenotypes in individual cells and analyze heterogeneous responses, thereby gaining a more comprehensive understanding of biological processes. Fluorescent labeling of proteins or staining of cells with fluorescent markers can allow for visualization of cellular and subcellular phenotypes. For example, fluorescent dyes or antibodies may be used to examine changes in cell shape under different conditions or perturbations. [1]High-content assays have become increasingly popular for investigating a broad range of biological processes, due in part to the emergence of automated microscopy, which has enabled researchers to analyze a wide variety of phenotypes. However, in order to obtain high-quality data from these assays, it is essential to carefully consider and optimize various imaging and analysis parameters.
Image acquisition is a critical component of the high-content assay workflow, and requires precise calibration of imaging settings such as illumination power, exposure time, and wavelength, in order to achieve the highest possible signal-to-noise ratio. Illumination correction and intensity decay correction are also essential for ensuring accurate and consistent results across fields and wells. Once images have been acquired, they must be processed and segmented to identify relevant objects and regions of interest. Raw image quality is an important consideration, and pre-processing techniques such as noise filtering and thresholding may be necessary to obtain optimal results. Object identification and analysis of properties for each population is then performed, with numerical data extracted from various readouts including morphological features, textures, and relationships between them. In order to effectively analyze this data, feature reduction and dimensionality reduction techniques such as supervised clustering are often employed. Multi-variate phenotype data can be visualized in various ways, including clustered network graphs, heat maps, or phenotype representations. Additionally, machine-learning approaches such as convolutional neural networks can be used for end-to-end feature extraction, enabling the identification and prediction of behavioral effects resulting from the perturbations included in the project.
In summary, high-content assays have become a valuable tool for studying a wide range of biological processes. Careful attention to imaging and analysis parameters is crucial for obtaining high-quality data, and the use of advanced techniques such as machine learning can further enhance the insights gleaned from these assays.
[1]M. Boutros, F. Heigwer, and C. Laufer, “Microscopy-Based High-Content Screening,” Cell, vol. 163, no. 6, pp. 1314–1325, 2015, doi: 10.1016/j.cell.2015.11.007.
n